Leveraging Custom MLOps for Superior Machine Learning Model Management
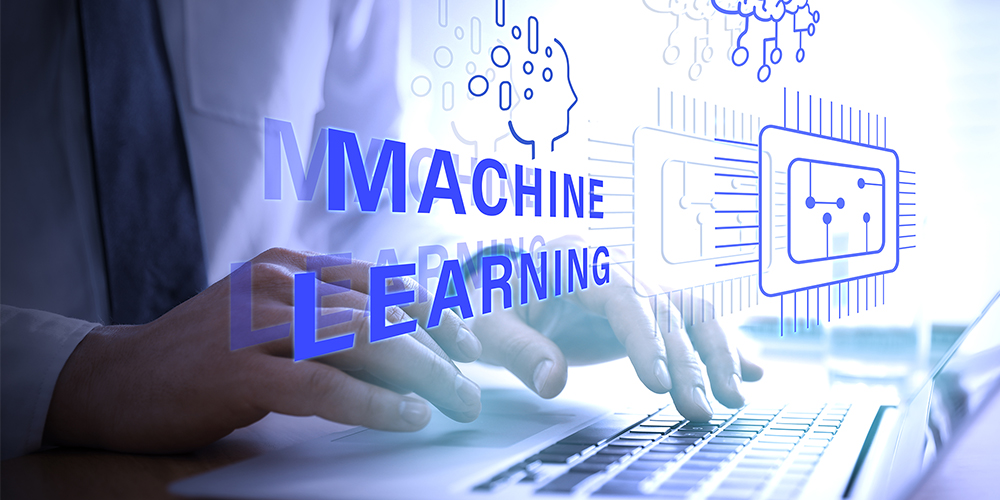
For today’s data-driven organizations, machine learning (ML) models are no longer a novelty but a strategic imperative. Sophisticated models can unlock transformative capabilities, from hyper-personalized customer experiences to next-level business process automation. However, the journey from cutting-edge model development to real-world impact is fraught with challenges. Here’s where custom MLOps emerges as a game-changer.
MLOps: Beyond the Buzzword
While the term “MLOps” has become ubiquitous, it’s crucial to delve deeper than the hype. Custom MLOps transcends a one-size-fits-all approach. It entails meticulously tailoring practices and tools to the specific needs and complexities of an organization’s unique model landscape. This nuanced approach is particularly valuable for highly-read audiences, who understand that a generic MLOps solution may not suffice for their advanced models.
Custom MLOps: A Competitive Advantage for the Discerning Leader
For the well-read leader, custom MLOps offers a distinct edge:
- Optimizing for Edge Cases: Custom MLOps caters to the specific requirements of cutting-edge models. For instance, a financial institution developing a bespoke fraud detection model can design a custom MLOps framework that prioritizes real-time responsiveness and robust security measures. According ACFE, 26% of organizations plan to adopt AI and ML for fraud detection in the next 2 years. Custom MLOps ensures this ROI is maximized by optimizing the model lifecycle for this specific purpose.
- Ensuring Explainability and Transparency: A highly-read audience is likely attuned to the growing emphasis on explainable AI (XAI). Custom MLOps can integrate XAI tools and techniques seamlessly, fostering trust and regulatory compliance. A recent McKinsey report highlights that 70% of businesses believe explainability is critical for successful AI adoption. Custom MLOps directly addresses this growing concern.
- Addressing Bias and Fairness: Custom MLOps empower organizations to proactively address potential biases in complex models. By incorporating fairness checks throughout the model lifecycle, custom MLOps ensures models are aligned with ethical considerations. Custom MLOps offers a powerful tool to mitigate this risk.
Beyond the Core: Customizing the MLOps Framework
The core components of an MLOps framework – architecture, tools, training, testing, deployment, and monitoring – remain essential. However, customization elevates these elements to a new level:
- MLOps Architecture: A custom architecture considers the specific needs of the organization’s model portfolio. For instance, an organization heavily reliant on deep learning models might prioritize containerization technologies like Docker for efficient deployment. The global market for containerization technologies is expected to reach $8.2 billion by 2025, highlighting its growing importance for complex model deployments.
- MLOps Tools: The vast MLOps toolset offers a buffet of options. Custom MLOps involves selecting and integrating the most suitable tools for each stage of the model lifecycle, ensuring a smooth and efficient flow. The MLOps market itself is projected to reach $5.9 billion by 2027, indicating the vast array of solutions available for customization.
Orchestrating the Symphony: CI/CD for Custom MLOps
Continuous Integration and Continuous Delivery (CI/CD) remains the cornerstone of automation in MLOps. However, custom MLOps takes CI/CD a step further. By meticulously tailoring CI/CD pipelines to the specific needs of each model, custom MLOps ensures seamless integration, testing, and deployment, even for highly complex models.
Beyond Deployment: Custom Monitoring and Maintenance
The well-read audience understands that a model’s journey doesn’t end at deployment. Custom MLOps goes beyond basic monitoring by incorporating advanced techniques like anomaly detection and federated learning. This ensures not only that models perform optimally but also that they continuously adapt to evolving data and real-world conditions.
Conquering Challenges: Custom MLOps as a Mitigation Strategy
While custom MLOps offers immense value, it doesn’t negate the inherent challenges of ML model management. However, it equips organizations with a powerful weapon in their arsenal:
- Data Deluge, Quality Control: Custom MLOps can integrate advanced data management tools to ensure the quality and consistency of data used or training and retraining complex models. Studies by MIT Sloan Management Review show that poor data quality costs organizations an average of 15-25% of their revenue. Custom MLOps help mitigate this risk by focusing on data quality throughout the model lifecycle.
- Governance Granularity: The nuanced approach of custom MLOps empowers organizations to establish robust governance frameworks tailored to the specific risks and regulatory requirements associated with their custom models. Gartner predicts that 75% of large organizations will have established AI governance frameworks by 2024. Custom MLOps ensure organizations stay ahead of this curve.
- Collaboration Cadence: Custom MLOps fosters a culture of collaboration by providing a common platform for data scientists, ML engineers, and operations teams to work seamlessly on complex models. The latest research suggests that fostering collaboration between these teams is critical for successful AI adoption. Custom MLOps directly addresses this need.
- Scalability Secured: Custom MLOps frameworks are designed with scalability in mind, ensuring they can accommodate the growing volume and complexity of an organization’s model portfolio. The number of machine learning models deployed in production environments is expected to grow by 400% by 2025. Custom MLOps ensures organizations are prepared for this exponential growth.
- Security Fortified: Custom MLOps integrates security best practices from the outset, ensuring models are protected from potential vulnerabilities and comply with relevant data privacy regulations. PwC found that data security breaches cost businesses an average of $1-20 million. Custom MLOps helps organizations safeguard their models and data from such threats.
In the ever-evolving landscape of machine learning, custom MLOps is not a luxury but a necessity for organizations seeking to maximize the return on their investment in advanced models. By meticulously tailoring MLOps practices to their specific needs, highly-read leaders can unlock the full potential of their models, achieving significant business benefits and staying ahead of the curve in the age of AI.
However, navigating the complexities of custom MLOps implementation can be a daunting task. This is where partnering with an experienced MLOps solutions provider can be invaluable. Integra, for instance, offers a comprehensive suite of custom model development services, including custom MLOps implementation. Our team of experts can help you design and deploy a custom MLOps framework that perfectly aligns with your unique model landscape and business goals.
By leveraging Integra’s expertise in custom MLOps, you can ensure your machine learning models deliver not just cutting-edge capabilities but also tangible business results. Take the first step towards maximizing the value of your AI investments and contact Integra today to explore their custom MLOps solutions.
News & Insights
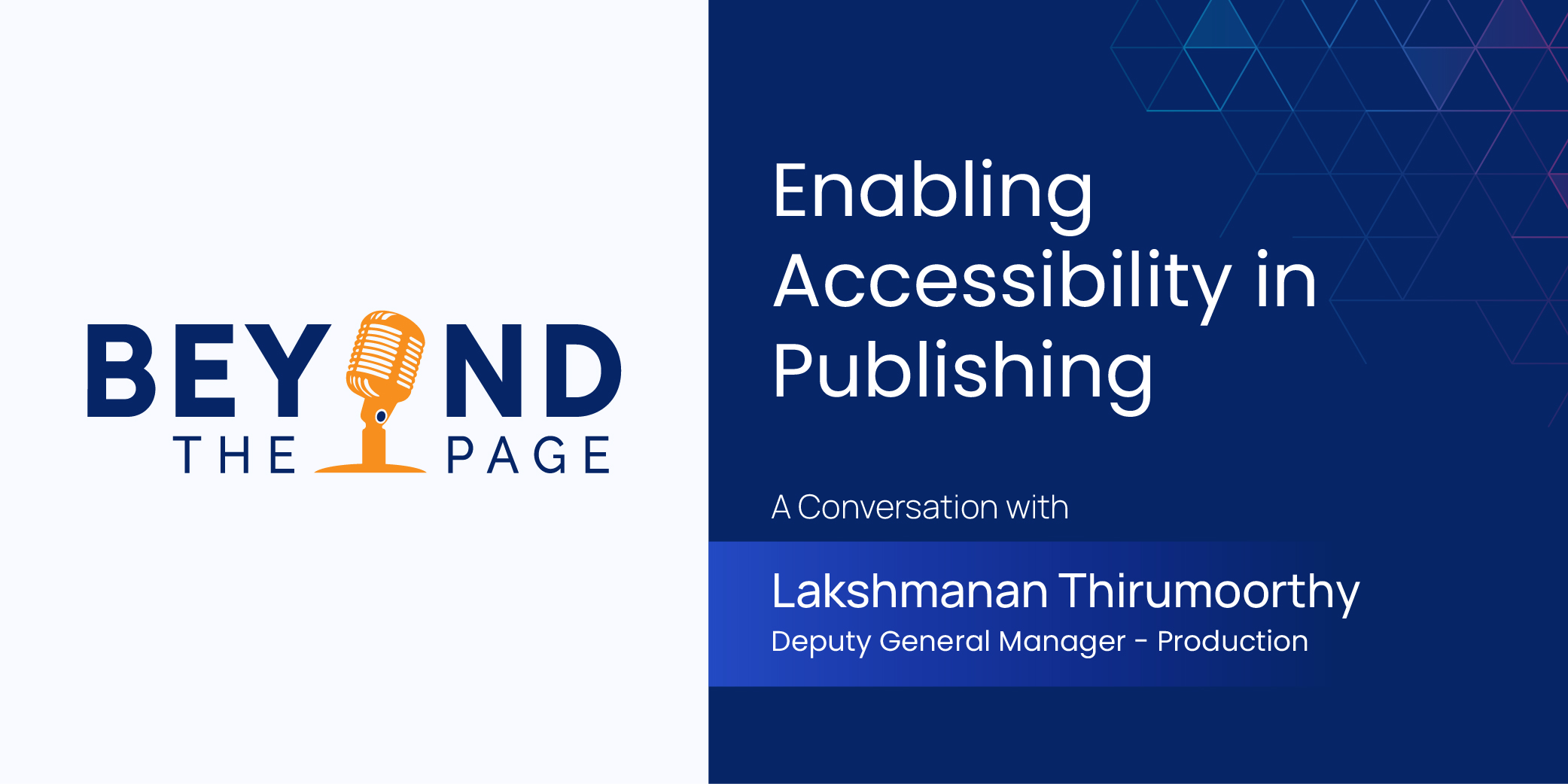
Enabling Accessibility in Scholarly Publishing – A Conversation with Lakshmanan Thirumoorthy
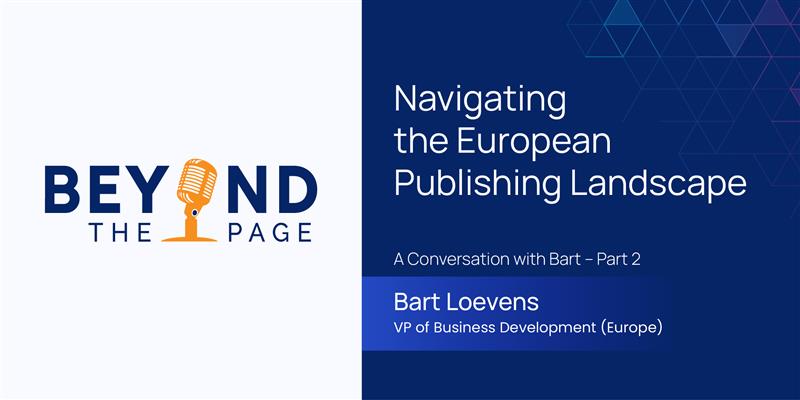
Navigating the European Publishing Landscape – A Conversation with Bart – Part 2
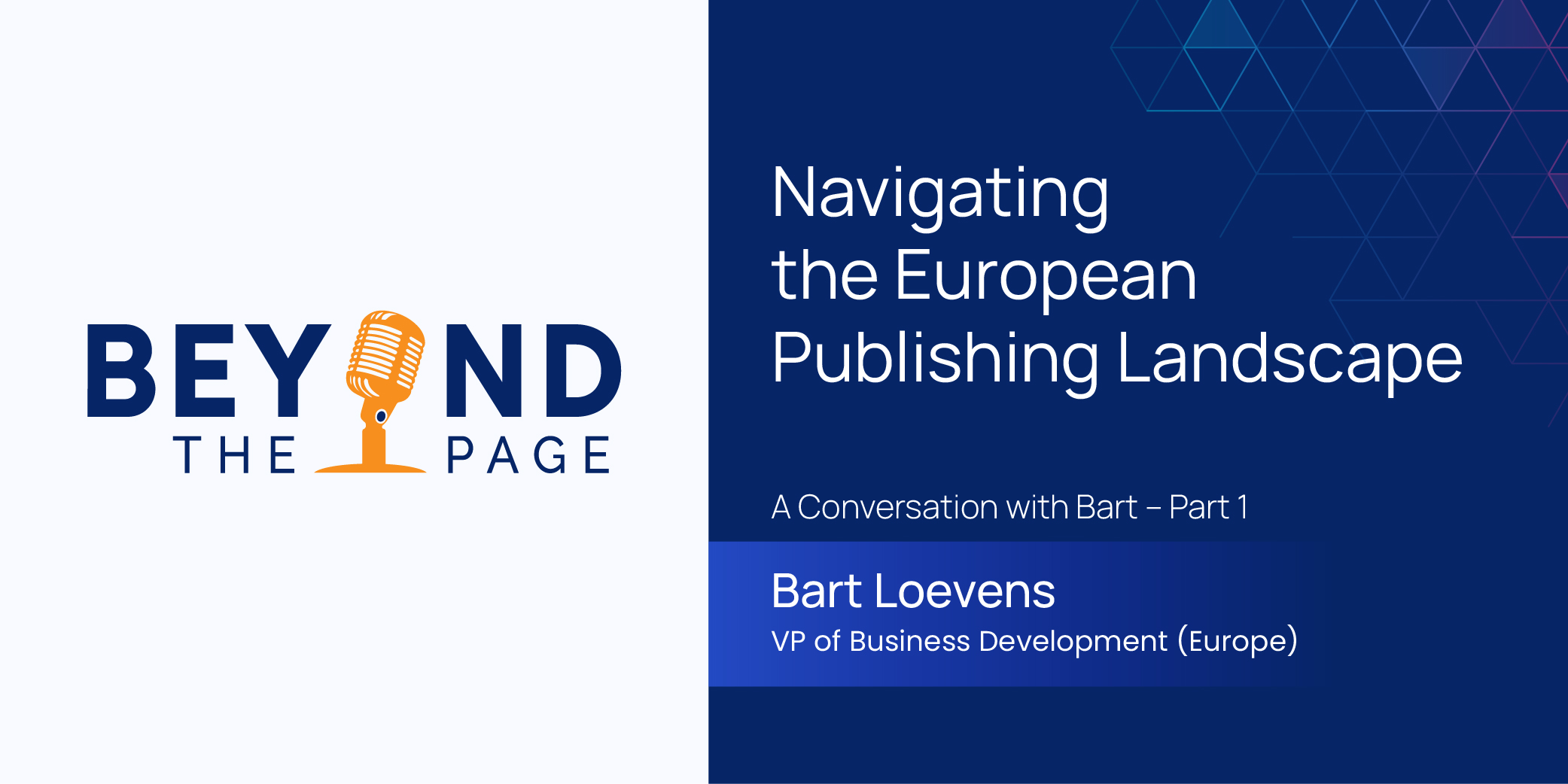